Information Finance
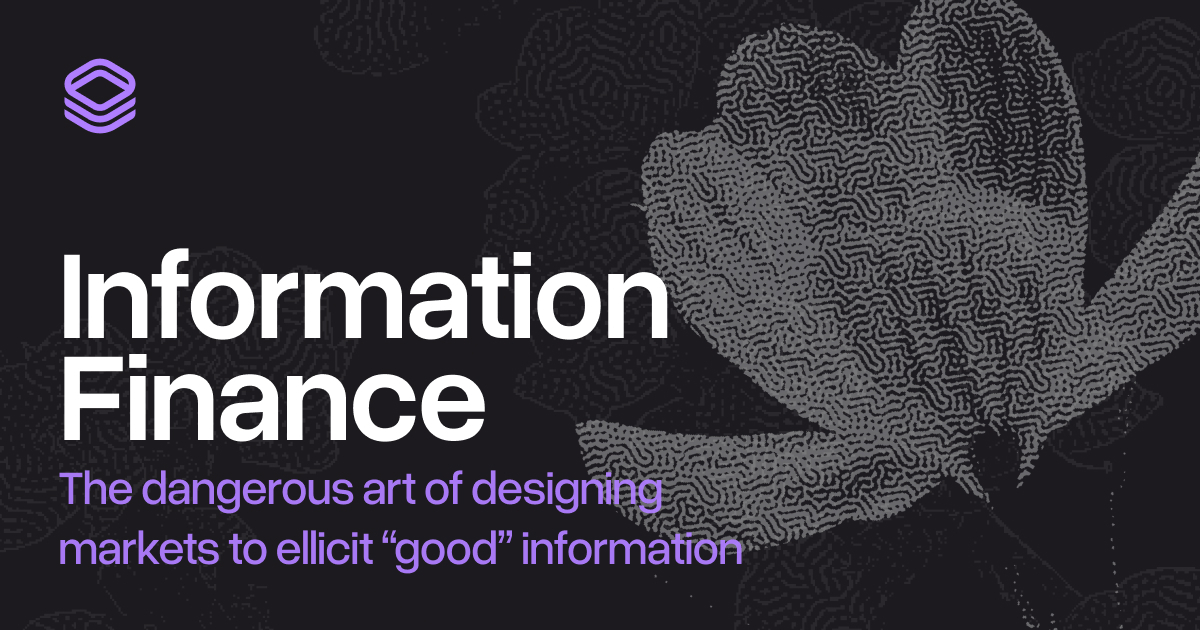
I.
Vitalik Buterin coined a term I’ll do my darndest to define. Information finance: the dangerous art of designing markets to elicit “good” information.
Buterin cites, as one example, prediction markets—an old idea with lots of baggage, but, perhaps, still with some utility. Buterin uses Venezuela’s 2024 election as an example: Polymarket priced the chance of González besting Maduro at a relatively high 23%, despite the seemingly low prior probability of Maduro being dethroned. The market’s probability turned out to be closer to correct: the opposition mounted a surprisingly competitive campaign.
This example forces a discussion on what we might mean by “good” information. Is it accuracy—the market's ability to surface probabilities closer to ground truth? Or is it the market's capacity to aggregate disparate signals that challenge our priors?
Consider a prediction market on climate outcomes. A market showing high probabilities of catastrophic warming might become self-negating by spurring policy action. Here, “good” information isn't just about accuracy—it's about catalyzing beneficial responses.
Buterin gestures toward this dichotomy when he discusses a point I’d never considered: markets can be combined to produce conditional markets, markets that could allow bettors to understand how an outcome may influence some future event. He uses the share price of a firm as an example:
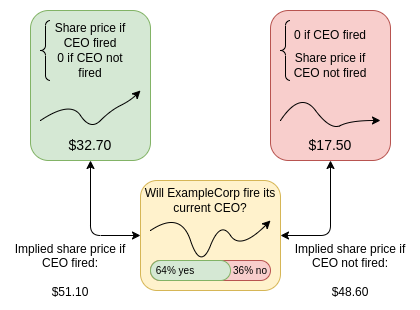
From Buterin. From prediction markets to info finance (2024)
Quoting Buterin again:
…you ask people to bet on (i) which decision will be chosen, (ii) value of M if decision A is chosen, otherwise zero, (iii) value of M if decision B is chosen, otherwise zero. Given these three variables, you can figure out if the market thinks decision A or decision B is more bullish for the value of M.
It also begs a deeper question: Can we distinguish between information that's “good” because it's correct and information that's “good” because it leads to better outcomes? Not in market terms alone, likely. But markets are culture, and, embedded in the right culture, I buy the premise that the right markets can help people make better decisions.
II.
Premise bought, we're brought to the next point: can these markets make decisions for us? The question is particularly salient for DAOs: voting takes time and energy, and many DAO members are under-engaged. Rightly designed, conditional markets could act as decision mechanisms.
Imagine a DAO deliberating on a protocol upgrade. Traditionally, DAO members would vote directly on the proposal. Imagine instead a prediction market: Traders could price shares representing “Protocol TVL will increase by >10% post-upgrade,” or “Block times will decrease to under 6s after upgrade.” The market prices would aggregate participants' beliefs about the upgrade's likely effects, potentially providing better signals than raw vote counts. Aggregating these signals could trigger an automatic upgrade once the aggregated signal exceeds a threshold.
This concept raises a thorny issue: Markets that directly determine decisions create feedback loops. Traders aren't just predicting outcomes—they're causing them. If a conditional market shows high confidence that a protocol upgrade will succeed, and that prediction automatically triggers the upgrade, the market incentivizes participants to buy “yes” shares, creating the conditions for their own success.
These feedback loops aren’t necessarily fatal. Traditional markets face similar dynamics: stock prices influence real business decisions, which in turn affect stock prices. (Soros made tens of millions of dollars on this principle—reflexivity, as he called it). For conditional markets, the solution might lie in market design. Multiple, interlinked markets that price different aspects of a decision, could make manipulation prohibitively expensive. Time delays between market signals and governance actions could allow prices to settle, better reflecting “good” information rather than the strategic incentives of market participants.
III.
Lots of questions, lots of problems to pursue. Two features make this topic relevant to Layer. First, conditional markets (hell, even regular prediction markets) will frequently require trusted, off-chain compute in order to get information about the outside world. Imagine a conditional market that tries to achieve some climate outcome. It will need to access off-chain state: satellite images, carbon emissions estimates, and so on. It may even need AI models to interpret some of that data (from the latest satellite images, estimate mangrove forest density; then, estimate change yearly). Here enters Layer. Through its AVSs, Layer allows conditional markets access to trustworthy off-chain compute, including AI.
Second, AI agents could act as market participants. AI agents could provide liquidity and help markets function more efficiently. They might even help surface "good" information by identifying subtle patterns in market data that human traders miss. But AI participation also introduces new risks. AI agents might learn to manipulate markets in ways that human traders can't detect or counter. They might develop strategies that optimize for market returns while undermining the very purpose of information finance: eliciting useful information about the world. Here, Layer also offers some assistance. AVS tooling could allow agents to run on-chain. Thus the agents’ models could remain (i) inspectable and (ii) accountable to governance–a check against these agents’ worst behaviors.
Designing any of these systems would not be easy. But the upside could prove worth the risk: properly designed, information finance could help solve some of our most pressing coordination problems. Conditional markets could help us understand and manage complex systemic risks, from climate change to AI development, without taxing traditional voting systems.
The key will lie in market design. We need markets that are robust to manipulation, that align incentives properly, and that produce information that's both accurate and useful. This might mean multiple layers of markets, each checking and balancing the others. It might mean careful constraints on AI participation, or new mechanisms for human oversight. It might mean embedding these markets in broader governance structures that can interpret and act on their signals appropriately.
IV.
Earlier, I characterized information finance as a “dangerous art.” It’s dangerous because, when information markets fail, they may do so spectacularly. It’s an art because its success lies not only in market design, but in designing cultures that can work with these markets. To use these markets, we need to foster cultures that can leverage their insights. Here, only active experimentation will do any good: cultures are made by doing.